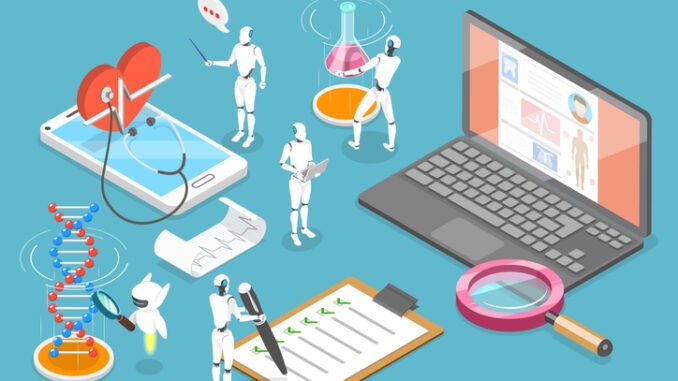
David Newey explores the risk of bias in AI health technology and shares what needs to be done to mitigate it
CREDIT: This is an edited version of an article that originally appeared on Digital Health
Artificial Intelligence (AI) relies on two key components; the development of an algorithm, and training data to predict outcomes. It is here that various factors can introduce bias and this can be introduced in a number of ways:
- Implicit bias: unconscious prejudice formed against person(s) that is not easily noticed by the holder of such prejudice.
- Sampling bias: a statistical problem where the sample data may be skewed towards specific sections of the data.
- Temporal bias: we can build a machine-learning model that eventually becomes obsolete due to future events not factored into the model.
- Over-fitting to training data: this is when AI models accurately predict values from the training dataset but cannot predict new data accurately, and is thus unrepresentative of the general population.
- Edge cases and outliers: outliers are data points not within the data’s normal distribution. Edge cases are errors (missing/incorrect datasets) or noise (additional, irrelevant datasets that could impact the machine learning process negatively).
Just like societal values, an AI algorithm is affected by temporal factors derived from:
- The demographic make-up of society affecting the team that develop it, and the training data used.
- The scientific knowledge and research from which the algorithm is derived
- That systems that are either modern enough, or have sufficient investment, to be able to incorporate AI algorithms
As a result, code written in 2022 could legitimately be out of date within five years or fewer, depending on the changes that occur in these factors over time.
How can this be addressed?
There is precedent for addressing these types of concern; the general data protection regulation legislation has provisions regarding the need to for organisations to provide transparency around the use of AI-based decisions and includes the ability for an individual to opt out of being subject to an AI-based decision that has legal, or similarly significant, effects.
Much like best practice derived from an information technology infrastructure library for the creation of application and data registers, it should now be incumbent on chief information officers to urgently look at their own digital ecosystems and put governance measures in place to both track and regularly review the AI algorithms in use within their organisations.
An AI oversight committee should be formed that regularly approves the use AI algorithms against set criteria which address applicability and bias, as well as setting ‘licenced use’ dates for software – after which it should either be re-licenced or taken out of service.
By being proactive at this stage, organisations can get ahead of the curve and be in the best possible place to comply with future legislation and regulation.
Be the first to comment